The relationship between anthropometric and metabolic risk factors and testicular function in healthy young men
Article information
Abstract
Objective
This study investigated the relationship of anthropometric and metabolic risk factors with seminal and sex steroidal hormone parameters in a screened population of healthy males.
Methods
The participants were healthy young men without chronic or congenital diseases. The body composition parameters that we investigated were measured weight, height, and waist circumference (WC), as well as bioelectrical impedance analysis. Semen samples were analyzed for semen volume, sperm concentration, sperm motility and morphology, seminal pH, and liquefaction time. Biochemistry analysis, including glucose and lipid metabolism parameters, was conducted on fasting blood samples. Testicular volume was calculated separately for each testis using ultrasonography.
Results
Body mass index exhibited an inverse association with total sperm count. WC showed negative correlations with numerous seminal parameters, including sperm concentration, total sperm count, sperm morphology, and follicle-stimulating hormone levels. The basal metabolic rate was associated with seminal pH, liquefaction time, and sperm motility. WC, fat mass percentage, and triglyceride levels exhibited negative correlations with sex hormone binding globulin. The measures of glucose metabolism were associated with a greater number of seminal parameters than the measures of cholesterol metabolism. C-reactive protein levels were inversely associated with sperm concentration and total sperm count.
Conclusion
Anthropometric and metabolic risk factors were found to predict semen quality and alterations in sex steroidal hormone levels.
Introduction
Sperm counts have been declining worldwide in recent decades, and infertility has become a serious health concern [1-4]. Male factor infertility has been identified in approximately 50% of infertile couples [5]. There has also been a parallel increase in chronic diseases and cancer. Studies have shown that men with poor semen quality are at risk for long-term morbidity and mortality [6-9]. Increased cancer and mortality rates related to lifestyle factors, obesity, and diabetes have been reported in men with impaired infertility [9,10], and there is growing evidence linking metabolic disturbances with male infertility. Obesity-related metabolic disorders, including metabolic syndrome, diabetes, hypogonadism, osteoporosis, and cardiovascular disease [11], may contribute to a potential decline in semen quality.
Metabolic syndrome, which is related to unhealthy dietary habits and a sedentary lifestyle, is also associated with poor general health and chronic systemic diseases. It is characterized by central obesity, dyslipidemia, insulin resistance, and hypertension [12]. In addition, both metabolic syndrome and male infertility have been associated with hypogonadism and high mortality risk [13,14]. Further studies have also shown that eugonadal men with a low sperm count had a higher risk for metabolic and cardiovascular diseases [13,14]. Body mass index (BMI), waist circumference (WC), and non-alcoholic fatty liver disease (the liver component of metabolic syndrome) have been associated with a decline in sperm output; however, the evidence regarding this point is still being debated and is far from conclusive [11].
Given the conflicting data and biological plausibility of this association, we conducted a screening study of healthy men. We investigated anthropometric and metabolic risk factors to identify predictive variables for seminal and sex steroidal hormone parameters.
Methods
1. Study population
This study was designed to measure the predictive associations between anthropometric, metabolic, hormonal, and seminal parameters in a population of healthy young men. Men being trained at a school for security services, arriving from different zones of the country, were included in the study. The participants underwent a thorough medical examination before acceptance to the school and had no chronic or congenital diseases, drug addiction, or anabolic steroid abuse. The study was approved by the Ethics Committee of Recep Tayyip Erdogan University (approval no. 224) and written informed consent was obtained from each participant.
All participants underwent a diagnostic workup with a thorough medical history that included comorbidities, a physical examination, anthropometric measurements, semen analysis, biochemistry assessment, and color Doppler ultrasound of the scrotum. For each participant, weight and height were measured, BMI was calculated, WC was measured, and bioelectrical impedance analysis (BIA) was performed using the Tanita BC-420MA (Tanita UK Ltd.). The physical examinations included a physical assessment for varicocele. Testis and epididymis size was measured by color Doppler ultrasound, and the volume of each testis was calculated using the following formula: testicular volume=[length (mm)×width (mm)×height (mm)]×0.71/1,000 (mL). Varicocele was identified by physical examination when the maximal venous diameter was >3 mm and increased with the Valsalva maneuver.
2. Assays
Fasting blood samples were drawn from all participants in the morning, between 9:00 AM and 12:00 PM. Serum sex steroidal hormones including testosterone (T), follicle-stimulating hormone (FSH), luteinizing hormone (LH), prolactin, and 17‐β‐estradiol were measured using a chemiluminescent microparticle immunoassay method with an Abbott Architect i2000 autoanalyzer (Abbott). The remaining metabolic parameters, including glucose and lipid metabolism, were measured by automated analyzers. The homoeostasis model assessment was calculated as fasting insulin (μU/mL)×fasting glucose (mmol/L)/22.5.
3. Semen analysis
Semen analysis was performed by two experienced biologists in the laboratory of the Andrology Unit. Semen samples were analyzed for semen volume, sperm concentration, sperm motility and morphology, seminal pH, and liquefaction time and were assessed according to the 5th edition of the World Health Organization (WHO) manual for human semen analysis. After 3 days of sexual abstinence, semen samples were collected by masturbation in a sterile container and were incubated at 37 °C during liquefaction. Subsequently, semen volume was estimated from the weight of the semen, assuming a sperm density of 1 g/mL, and was calculated by the gravimetric method. A Neubauer Improved hemocytometer (Shanghai Qijing Biochemical Instrument Co., Ltd.) was used to count the number of sperm (106/mL), and the total sperm count was calculated. Progressive motility was measured (WHO grades A+B) and morphology was assessed according to the Kruger strict criteria.
4. Statistics
1) Preprocessing the dataset
The R programming language (R Foundation for Statistical Computing) and SPSS version 23.0 (IBM Corp.) were used for statistical analysis. All quantitative data were summarized as minimum/maximum or arithmetic mean and standard deviation. While seminal and hormonal parameters were dependent variables in the model, metabolic and anthropometric variables were independent variables. Metabolic and anthropometric variables were used to predict the hormone concentrations and testicular volume by separately measuring the right testis and the left testis. Variations in the dependent variables were explained by the independent variables as estimated by multivariate linear regression analysis. There were no outliers in the data set. However, multiple normal distributions were not met with the Anderson-Darling test (Figure 1). Although data for the variables disrupting the multiple normality were transformed, the multiple normality assumption still could not be achieved (Figure 2).
2) Multilayer perceptron model
The multilayer perceptron (MLP) model, which is the most widely used artificial neural network model and does not require normality assumptions, has been deemed appropriate for use in statistical predictions [15]. The MLP is a feedforward model that maps input datasets to an appropriate output set by adjusting the weights between internal data nodes. Since multiple normality assumptions were not met, we used a correlation matrix to select the variables to be used in the MLP model. For the correlation matrix, Spearman rank correlations were examined between the seminal and metabolic variables, and between the hormonal and metabolic parameters. Independent variables with the highest correlations to the dependent variables were included and analyzed in the MLP model.
The data set was divided into two divisions, training (80%) and testing (20%), and the sum of the squares error (SSE) and relative error (RE) values were checked to assess model performance. In addition, the variables that influenced the seminal or hormonal parameters were determined by their importance and relative importance values (relative importance >50%). The extent to which the independent variables could explain the change in dependent variables was evaluated by the lowest SSE and RE metrics.
3) Power analyses
A sample size calculation (power analysis) was conducted. If there are six or more independent variables in an estimation model with dependent and independent variables, the minimum sample size is obtained with the formula n=104+k (k=number of independent variables). In this study, because the sample size required for each group was 120 (5×24) or 5 times the number of variables, and was still 130 (104+24) when the number of variables was 6 or more, the sample size was considered sufficient.
Results
Of the 304 cohort members invited to participate in the study, 121 (39.8%) men provided a semen sample and underwent all analyses including a physical examination, biochemistry assessment, and testicular ultrasound. All participants had college degrees and similar socio-economic status (data not shown). The mean age of the participants was 27.9 years (range, 22 to 32), none were married, and 69 (57%) and 19 (15.7%) were smokers and consumers of mild alcohol, respectively. No participants had cardiovascular, metabolic, congenital, or chronic diseases. Varicocele (grade 2+3) was detected by physical and ultrasound examinations in the left testicle of 23 men (19%), and subclinical varicocele was observed in 10 (8.2%) and six (4.9%) men in the left and right testicles, respectively. Semen analysis revealed normospermia in 101 (83.5%) participants. Azoospermia was not detected. Metabolic syndrome was observed in three participants (2.5%) based on the National Cholesterol Education Program-Adult Treatment Panel III definition (2001). The characteristics of the participants are presented in Table 1.
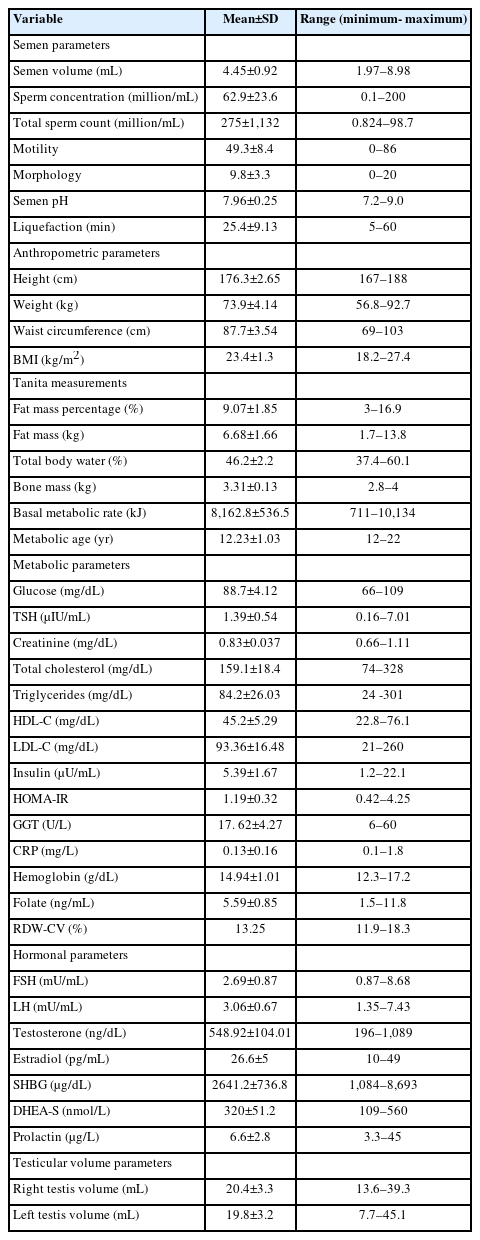
The variables used to evaluate the relationship of anthropometric and metabolic risk factors with testicular function
Changes in the seminal and hormonal parameters (dependent variables) explained by the metabolic or anthropometric parameters (independent variables) are shown in Table 2 and 3, respectively. The highest percentage of explanation performance for seminal parameters in the training data set was found for liquefaction time (SSE=35.04, RE=0.694), and in the testing data set for sperm count (SSE=3.831) and liquefaction time (RE=0.692) (Table 2). However, the highest explanatory performance for hormonal and testicular parameters was found for LH (SSE=30.12, RE=0.76), and right testis volume (SSE=5.61) and testosterone (RE=0.76) in the training and testing data sets, respectively (Table 3).
Discussion
The associations observed in this study showed that WC may be a more useful measure for male infertility than BMI. Although BMI was associated with total sperm count, WC was additionally predictive of sperm concentration and morphology. However, basal metabolic rate (BMR) was observed as the only predictive anthropometric parameter for seminal pH, liquefaction time, and sperm motility. In terms of metabolic parameters, the measures of glucose metabolism were associated with a greater number of seminal parameters than cholesterol metabolism.
BMI has been identified as a risk factor for male infertility in several studies. However, conflicting results have been reported for numerous methodological reasons [16]. In addition, WC has been associated with a decline in semen volume [17-19], sperm concentration [19,20], total sperm count [17,18,21], sperm morphology [20], and sperm motility [14,18,19]. The studies were largely performed in infertile men whose testicular function was already impaired [17], and differences in the studies can be explained by variance in the study group proportions [17]. In addition to its associations with seminal parameters, WC was also a negative predictor of FSH, the most prominent hormone in spermatogenesis. In this study, BIA measurements were extensively studied for seminal and hormonal parameters. BIA is widely used for estimating body composition [22] and is a more accurate measure of adiposity than BMI. Adiposity is strongly associated with obesity-related health problems [23]. The BMR or daily minimum level of energy utilized by a body in a physical and psychological resting state [24], was found to be the only BIA measurement predictive of seminal pH, liquefaction time, and sperm motility. Although BMR may be an indirect indicator of energy expended during sperm motility, in this study a positive prediction was observed between testicular volume and testosterone. Androgen deficiency was associated with changes in whole-body metabolism including decreased rates of body protein turnover and decreased resting energy expenditure [25]. BMR decreases by 1% to 2% every 10 years, and testosterone levels fall at an average of 1.6% per year [26,27]. Like testosterone, BMR is related to the metabolic rate of tissues [25-27] and could be used as an indicator in the clinical evaluation of patients with hypogonadism. Further studies of this relationship are needed.
Cholesterol is the precursor of sex steroidal hormones; however, little is known about the relationship of cholesterol and lipid metabolism with seminal parameters. In this study, both cholesterol and glucose metabolism were found to explain the variance in seminal parameters. Preclinical models confirm our findings that a high-fat diet may result in decreased sperm motility, sperm count, and morphology, and in increased sperm cholesterol content [28,29]. In this study, WC, fat mass percentage and triglyceride level were negatively predictive of sex hormone binding globulin (SHBG). Lower levels of SHBG have been linked to obesity [30]. Obesity has been shown to affect the gonadotropin-releasing hormone-LH/FSH pulse, which may deteriorate testicular steroidogenesis and spermatogenesis [31]. Metformin, an oral insulin-sensitizing agent that improves insulin sensitivity, has been used in human and animal models and resulted in improvements in seminal parameters [32] and in obesity-induced poor spermatogenesis [33]. Insulin resistance and dyslipidemia are associated with oxidative stress [34], and increased levels of reactive oxygen species impair germ cell production and sperm motility and give rise to sperm DNA damage [35]. Apart from our findings on lipid and glucose metabolism, we found that serum levels of C-reactive protein negatively predicted sperm concentration and total sperm count. Chronic inflammation disrupts the hypothalamic–pituitary-testicular axis and may result in poor semen quality [36].
This study had limitations. Because the cohort included unmarried healthy young men without chronic and genetic diseases, selection bias was possible. Therefore, it is difficult to generalize the results to other male populations (e.g., men with infertility or advanced age). Although the cohort was recruited from a single academic outpatient clinic, the subjects participating in this study came from multiple parts of the country, and thus can be considered to represent the whole country. Only one semen sample was obtained per subject; nevertheless, it has been reported that one sample is adequate to evaluate semen quality in epidemiological studies [37]. Differences in the predictive variables between right and left testis volume may be due to volume differences, the fact that varicocele was more common on the left side, or by chance. Finally, the cross-sectional design does not permit inference of a causal relationship between metabolic and seminal parameters.
In conclusion, this study demonstrated that anthropometric and metabolic parameters have predictive relationships with seminal parameters and sex steroidal hormones. These findings add further insight into the underlying mechanisms of these relationships, which can be used to develop preventive and therapeutic interventions that improve semen quality in the management of infertile men.
Notes
Conflict of interest
No potential conflict of interest relevant to this article was reported.
Author contributions
Conceptualization: HU, MH. Data curation: HU, MH, EZ, YÖO, BS, GA. Formal analysis: HU, MK. Methodology: HU, MH, EZ, YÖO, BS, GA. Writing-original draft: HU. Writing-review & editing: HU.