Differential gene expression analysis of human cumulus cells
Article information
Abstract
Objective
This study was performed to explore the possibility that each oocyte and its surrounding cumulus cells might have different genetic expression patterns that could affect human reproduction.
Methods
Differential gene expression analysis was performed for 10 clusters of cumulus cells obtained from 10 cumulus-oocyte complexes from 10 patients. Same procedures related to oocyte maturation, microinjection, and microarray analyses were performed for each group of cumulus cells. Two differential gene expression analyses were performed: one for the outcome of clinical pregnancy and one for the outcome of live birth.
Results
Significant genes resulting from these analyses were selected and the top 20 affected pathways in each group were analyzed. Circadian entrainment is determined to be the most affected pathway for clinical pregnancy, and proteoglycans in cancer pathway is the most affected pathway for live birth. Circadian entrainment is also amongst the 12 pathways that are found to be in top 20 affected pathways for both outcomes, and has both lowest p-value and highest number of times found count.
Conclusion
Although further confirmatory studies are necessary, findings of this study suggest that these pathways, especially circadian entrainment in cumulus cells, may be essential for embryo development and pregnancy.
Introduction
The prevalence of infertility is increasing, and new treatment options have been developed and collectively termed assisted reproductive techniques (ARTs) [1,2]. One of the most frequently used ART method is intracytoplasmic sperm injection (ICSI). A very important step in human ARTs is noninvasive oocyte selection, followed by transfer of appropriate embryos because conducting this step properly reduces the risk of multiple gestations and increases the probability of pregnancy and live birth [3]. However, the morphological parameters used for embryo selection are not always efficient because embryos that have good morphology may not always have normal chromosomes [4].
Cumulus cells (CCs) are the follicle cells surrounding the oocyte in cumulus-oocyte complexes [5], which play an active role in the development process of oocytes [1]. Therefore, studies of cumulus-oocyte complexes may yield valuable insights into pregnancy and live birth mechanisms. Moreover, as CCs are considered to be waste tissue, use of these cells is not restricted by ethical considerations. For this reason, they are an ideal material for noninvasive methods to determine markers of oocyte and embryo quality in ICSI and to predict potential success in terms of clinical pregnancy and live birth in humans. Ample evidence exists that successful follicle maturation in the mammalian ovary involves efficient paracrine communication between oocytes and granulosa cells (GCs), and good paracrine communication requires appropriate genetic control. CCs have the potential to predict oocyte competence. Thus, the expression profiles of CCs may be used as a parameter to facilitate optimal embryo selection [4], and may therefore be related to pregnancy and live birth outcomes. Recent reports have provided a plethora of information about GC morphology and/or their genetic expression profiles related to ARTs. Gene expression microarray analyses are used for mRNA profiling [6], and this technique has been applied to predict differences in genetic expression between CCs grouped according to various parameters of oocyte and embryo competence. However, a disadvantage of analyzing grouped CCs is that pooled genetic information is analyzed using microarray sequencing, rather than CCs belonging to a specific oocyte.
Enrichment of the transcriptome analysis results of CCs could provide information about critical cellular mechanisms involved in clinical pregnancy and live birth, potentially facilitating the development of novel ARTs and therapies. This study was performed to explore the possibility that specific oocytes and their surrounding CCs might have different genetic expression patterns that could affect human reproduction. To this end, this study sought to identify differentially expressed genes in cases of clinical pregnancy and live birth in comparison to non-pregnancy and stillbirth, respectively. These results were further enriched by pathway analysis using the PANOGA tool, providing valuable insights into the cellular mechanisms involved in clinical pregnancy and live birth, which may be possible to exploit in order to predict the outcomes of clinical pregnancy and live birth after embryo transfer.
Methods
This prospective case series included 10 women (age, 23–35 years) who were referred to Ege University Medical Faculty Hospital for idiopathic infertility and received ART therapy. The total sample size was 10 oocyte-cumulus complexes obtained over a period of 14 months between October 2011 and December 2012. CC gene expression in 10 clusters of CCs obtained from 10 cumulus-oocyte complexes from 10 patients was individually evaluated by microarrays using a NimbleGen human gene expression 12×135K microarray kit (Roche, Penzberg, Germany). Although very few CCs were associated with each oocyte, each oocyte from which a transferred embryo developed was analyzed individually, along with its surrounding CCs, through microarray analysis. The results were reported using clinical pregnancy and live birth as outcome variables. This study included only patients with unexplained infertility, and excluded cases of infertility based on male factors and other female factors in the standard patient population. All participating patients provided oral and written consent. Approval for this study was granted by the Ethics Committee for Human Research of Ege University (11-2, 9; 28.03.2011). All procedures were applied in accordance with the ethical standards described in the 1964 Declaration of Helsinki and its later amendments.
1. Ovarian stimulation protocol and oocyte pick-up
Ovarian stimulation therapy was applied to women prior to ICSI. Recombinant follicle-stimulating hormone (FSH, 75–225 IU; Gonal F, Serono International, Geneva, Switzerland) was administered according to the patient’s ovarian response. Then, 0.1 mg of a gonadotropin-releasing hormone (GnRH) analogue (Decapeptyl; Ferring, Kiel, Germany) was started on the 21st day of the previous menstrual cycle. Transvaginal ultrasonography was performed at regular intervals during induction, and blood estradiol and progesterone levels were measured in order to assess follicular development. Human chorionic gonadotropin (hCG, 5,000–10,000 IU; Ovitrelle, Merck-Serono, Rome, Italy) was administered when the follicles achieved a sufficient diameter. After 34 to 36 hours, follicles were aspirated transvaginally into sterile tubes under anesthesia [7].
2. Evaluation of oocyte quality and collection of CCs
Cumulus-oocyte complexes were incubated in an incubator at 37°C in an atmosphere containing 5% CO2. CCs were dissected from cumulus-oocyte complexes using a sterile pipette and immediately transferred into a sterile tube individually. The CCs surrounding the same oocyte were taken as individual groups, and were not pooled together. The CCs were then stored at −80°C for further analyses for about 3 months [8]. The remaining CCs adjacent to the oocytes were removed from the oocytes with enzymatic and mechanical methods using a sterile pipette and hyaluronidase (Hyase, Vitrolife, Frolunda, Sweden). Oocytes were evaluated morphologically and according to their nuclear maturation.
3. ICSI and fertilization
Approximately 30 to 60 minutes after denudation, mature oocytes were subjected to microinjection procedures. After microinjection, all oocytes were incubated in a fertilization medium using an incubator at 37°C with an atmosphere of 5% CO2 (individually, not pooled). After 18 hours, the morphology of the pronuclei was evaluated to determine whether fertilization of the oocytes had occurred. Normal fertilization was defined as the presence of two pronuclei, with the first and second polar bodies and cytoplasmic localization of the polar bodies.
4. Embryo transfer and monitoring of pregnancy
About 20 hours after fertilization of an egg, the first mitotic division began and two-cell embryos were formed. Starting then, the embryos were evaluated according to the shape, size, and number of blastomeres, rate of fragmentation, rate of multinucleation, and degree of compaction [9,10]. On day 4 after fertilization, developing embryos were evaluated for compaction, morulation, and blastocoel formation. The inner cell mass and trophectoderm cell mass were evaluated for the compaction and cavitation processes of blastomeres. On day 4 after fertilization, laser-assisted hatching was applied to the embryos, and embryo transfer was performed. Beta-hCG levels in the blood of the patients were measured to determine pregnancy 12 to 14 days after embryo transfer. The patients were monitored to determine whether there was a gestational sac and to measure the fetal heart rate with ultrasound.
5. Microarray protocol
Gene expression in CCs was individually evaluated by microarrays in 10 clusters of CCs obtained from 10 cumulus-oocyte complexes from 10 different patients using a NimbleGen human gene expression 12×135K microarray kit according to the manufacturer’s instructions.Twelvesample hybridization was performed on a single microarray slide, with three probes per gene. Total RNA was isolated using an RNeasy kit (Qiagen, Hilden, Germany) according to the manufacturer’s instructions. cDNA synthesis from total RNA was carried out using a Superscript Double Chain cDNA synthesis kit (Invitrogen Life Technologies, Carlsbad, CA, USA). cDNA was marked using a single color DNA labeling kit (Nimble-Gen). cDNA was hybridized using the NimbleGen Hybridization System. Washing and drying of the array were carried out with a NimbleGen MS 2000 microarray scanner, and raw probe expression data were collected using DEVA Software (Roche Nimblegen, Meylan, France).
6. Microarray data analysis
Microarray data resulting from the sequencing of 10 CCs, each surrounding an oocyte from one of 10 different patients, were analyzed to identify differentially expressed genes associated with the dichotomous outcomes of clinical pregnancy (pregnant vs. nonpregnant) and live birth (livebirth vs. stillbirth). Two differential gene expression analyses were performed: one for the outcome of clinical pregnancy and one for the outcome of live birth. Data were preprocessed using the oligo package [11] in R (www.R-project.org, 2016) and statistical analyses for differential gene expression analyses were performed using the limma package [12] in R. Several probes can be used to detect the transcriptome level of a single gene. In such cases, we used the probe with the most significantly differentiated value of significance for that gene. Significant genes resulting from these analyses (with p<0.05) were selected and input into the PANOGA tool [13] for pathway analysis.
In order to determine the functionally enriched pathways of genes, PANOGA tool first aims to determine active subnetworks associated with those genes and their interactions via a protein-to-protein network. The BioGrid 3.4.141 protein-protein interaction (PPI) network [14] is loaded into Cytoscape [15] and the list of affected genes and their p-values are mapped onto the PPI network. To determine the active subnetworks of interacting proteins that are possibly associated with a disease, greedy search implementation of JActive Modules [16] is used. For functional enrichment of subnetworks, PANOGA tool computes the proportion of genes in a subnetwork that are also found in a human Kyoto Encyclopedia of Genes and Genomes (KEGG) pathway, compared to the overall proportion of genes described for that pathway. For this purpose, ClueGO [17] plugin of Cytoscape is utilized. ClueGO works by facilitating a biological interpretation through visualization of functionally grouped terms in form of networks and charts. In order to determine statistical significance of an enrichment of the identified subnetwork, two-sided (enrichment/depletion) test based on hypergeometric distribution is applied, and the resulting p-values are corrected using the Bonferroni method for multiple testing.
Since PANOGA involves optimization procedures and stochastic processes, it was run 20 times for each case, and resulting KEGG [18] pathways were summarized across all runs for the outcomes of clinical pregnancy and live birth. In our analysis, we reported KEGG pathway ID, KEGG pathway name, significance of each pathway, pathwayrelevant genes that are found inthe original PANOGA input, and number of times each pathway found. The more frequently a pathway is found in an analysis, the less likely it is to be a random occurrence.
Results
Clinical pregnancy rates and live birth rates were used to assess the success of ICSI. Clinical pregnancy was observed in three of the 10 patients, and live birth occurred in two patients (Table 1). A total of 4,371 significant genes for clinical pregnancy and 1,975 significant genes for live birth were enriched to 152 and 151 KEGG pathways, respectively, through 20 PANOGA runs. The top 20 affected pathways for clinical pregnancy and live births are summarized in Tables 2 and 3, respectively. The two outcomes had 12 pathways in common, with different levels of significance, showing the involvement of certain cellular mechanisms in both clinical pregnancy and live birth. The 12 pathways that were significantly affected for both outcomes were: circadian entrainment, complement and coagulation cascades, Rap1 signaling pathway, morphine addiction, pathways in cancer, Ras signaling pathway, focal adhesion, cholinergic synapse, ErbB signaling pathway, chemokine signaling pathway, retrograde endocannabinoid signaling, and apoptosis. Circadian entrainment had a lower p-value (6.04×10–30) than other pathways. The pathways that were different between live birth and clinical pregnancy were primarily several signaling pathways involved in neurological signal trafficking. Unique clinically-relevant pathways include proteasome and endocytosis.

The laboratory and clinical results of 10 patients with unexplained infertility who underwent assisted reproductive technologies and whose cumulus cells underwent microarray analysis
We also conducted analyses of genes associated with both outcomes, and the results for the top 20 affected genes are summarized in Tables 4, 5 for clinical pregnancy and live birth, respectively. None of the top 50 significant genes for either outcome is in common. The most distinct significantly differentially expressed genes from both analyses that were also directly involved in the most affected pathways are reported below.
In clinical pregnancy, PRKCAB ranks 16th among the most significantly altered genes, and is found in 13 of the top 20 significantly affected pathways. COL4A ranks 7th among the most significantly altered genes, and takes part in two of the top 20 significantly affected pathways. FGG and C4BPA rank 21st and 24th, respectively, among the most significantly altered genes, and are also found in the complement and coagulation pathway. SLC8A2 ranks 27th among the most significantly altered genes, and plays a role in the calcium signaling pathway.
In the live birth transcriptome analysis, BDNF ranks 10th among the most significantly altered genes, and is found in two of the top 20 significantly affected pathways. NR4A3 ranks 2nd among the most significantly altered genes, and is found in the transcriptional misregulation in cancer pathway. ENDR ranks 22nd among the most significantly altered genes, and plays a role in pathways in cancer. MAGI1 ranks 26th among the most significantly altered genes, and is also found in the RAP1 signaling pathway.
Since our method maps the significant genes to a PPI network and finds active subnetworks involving many of the affected genes, it also takes into account the indirect effects of genes through cascades of interactions with certain cellular processes. Therefore, some of the genes might not be directly involved in the affected pathway, but instead may affect some of the genes in that pathway through interactions of proteins.
With this in mind, we investigated distances between genes within the PPI network for the top 20 significant genes resulting from differential expression analysis and for genes associated with the most significant pathways of both outcomes; circadian entrainment for clinical pregnancy and proteoglycans in cancer for live birth. Distance reported herein is the number of proteins in the PPI network in between two interested gene.
In circadian entrainment pathway, cumulative average distance over all genes is found to be 3.27. CALML3 is found to be the gene with lowest average distance of 2.6 and MTNR1B is found to be the gene with highest average distance of 4.
In proteoglycans in cancer pathway, cumulative average distance over all genes is found to be 2,91. TP53 is to be the gene with lowest average distance of 2.07 and IL12B is found to be the gene with highest average distance of 4.46. In pathway analysis, we focused only on the top 20 pathways ranked by statistical significance. The relevance of these pathways to clinical pregnancy and live birth was established via a thorough literature search, which is summarized in the Discussion section.
Discussion
1. Clinical pregnancy
Our analysis determined that circadian entrainmentis the most significantly affected pathway for clinical pregnancy. The affected genes and their interactions in circadian entrainment pathway are shown in Figure 1. Circadian entrainment is stabilized by the maintenance of regular light/dark and sleep/wake cycle and plays a critical role in steroid biosynthesis, including luteinizing hormone (LH) and GnRH secretions in pregnancy. It acts as a regulator of many bioprocesses, with the circadian clock regulating the timing of ovulation and progesterone secretion in the GCs and luteal cells of ovarian follicles. Disruption of the female circadian clock cycle has a negative effect on women becoming pregnant [19]. Melatonin also has an impact on reproduction, especially during pregnancy, by protecting against molecular damage and cellular dysfunction [20].

Significantly altered genes in the circadian entrainment pathway and their interactions for clinical pregnancy, denoted by red pathway nodes. Data from Kanehisa Laboratories. It is permitted to be used for academic purposes. Full definitions and descriptions for the pathway nodes can be found at https://www.genome.jp/kegg-bin/show_pathway?map=hsa04713&show_description=show.
The complement and coagulation cascades are other pathways that showed a significant difference between CCs depending on whether clinical pregnancy occurred. In a previous study that compared gene expression in CCs and GCs surrounding oocytes, it has been reported that there were differentially expressed genes related to the complement and coagulation cascades between these groups of cells [21]. Proteasomal proteolysis has multiple functions in the process of oocyte meiosis resumption and in other steps of nuclear maturation [22,23]. The results of current study also indicate that proteasome pathway might impact clinical pregnancy outcomes. Previous studies have shown that RAP1 signaling pathway, RAS signaling pathway, and ErbB signaling pathway have an impact on reproduction [24,25]. The RAS-mediated ERK1/2 pathway in pituitary cells, which is affected by elevated GnRH, is crucial for stimulating the LH surge and ovulation [26]. In current study, it is also demonstrated that RAP1 signaling pathway, RAS signaling pathway, and ErbB signaling pathway may play active roles in clinical pregnancy. Furthermore, genes related to morphine addiction, cancer, calcium signaling, and endocytosis pathways demonstrated significant differences in expression in CCs depending on whether clinical pregnancy was achieved. The presence of opioid receptor subtypes has been demonstrated in preimplantation embryos and in oviduct, and these have active roles in embryo development [27]. The hippo tumor suppressor pathway has functions in follicle cells related tooocyte polarity and development of the body axes in Drosophila [28]. Gonadotropin paracrine factors induce Ca2+ mobilization in CCs, which affects gamete development and function, and therefore fertilization and reproduction [29]. Furthermore, rat GCs may utilize FSH for biological processes through endocytosis [30].
Genes involved in neurological signaling pathways, such as glutamatergic synapse, cholinergic synapse, dopaminergic synapse, gamma-aminobutyric acid (GABA)-ergic synapse, long-term potentiation, and neuroactive ligand-receptor interaction pathways show significant differences in CCs depending on whether clinical pregnancy occurred. These pathways and related genes may play functional role in pregnancy, as they affect gonadotropin hormone secretion by regulating hormonal signaling pathways. Genes responsive to neurotransmitters, such as dopamine, glycine, and GABA may play important roles in folliculogenesis and oocyte maturation [31]. GABA is important for regulation of reproduction via GnRH neurons [32]. Genes that encode receptors for GABA and glutamate have been determined to be related with oocyte maturation [33]. In a recent study, significant differences have been reported in dopaminergic synapse, mitogen-activated protein kinases (MAPK) signaling, steroid hormone biosynthesis, steroid biosynthesis, GnRH signaling, oocyte meiosis, progesterone-mediated oocyte maturation, calcium signaling, and G-protein coupled receptor signaling pathways in goose ovarian tissue across different reproductive periods [34]. With the induction or inactivation of the LH choriogonadotropin receptor, the neuroactive ligand–receptor interaction pathway led to changes in the menstrual cycle, LH levels, ovarian size, and response to LH and hCG [33].
2. Live birth
In this microarray analysis of human CCs, significant differences are observed in genes related to several hormonal, metabolic, apoptotic, and neuronal pathways. These pathways may be associated with live birth, as shown by their differential expression according to whether live birth occurred.
In this study, genes related to proteoglycans in the cancer pathway, apoptosis pathways, tumor necrosis factor (TNF) and MAPK pathways, retrograde endocannabinoid signaling, and the transcription factor nuclear factor-κB shows a significant association with success of live birth. CCs and GCs synthesize proteoglycan by gonadotropin stimulation to produce an elastic matrix with mucus, and the synthesis of proteoglycan changes during follicular development and atresia [35]. Proapoptotic and antiapoptotic pathways in GCs are very importantin ovarian follicles and affect reproductive outcomes of various mammals, including humans [36,37]. During mammalian ovulation, TNF maintains apoptosis at the ovarian surface epithelium to promote stigma development and ovulation [38]. In a recent microarray analysis of gene expression in human GCs and CCs, it has been shown that TNF has been highly expressed in GCs [21]. Jun N-terminal kinase, p38 MAPK, and ERK signaling pathways coordinate proapoptotic and antiapoptotic factors in GCs to regulate follicular atresia [39]. The maturation of the mouse cumulus-oocyte complex depends on MAPK pathway in CCs [40]. Cannabinoid receptors are expressed in the human ovarian medulla and cortex [41]. The endocannabinoid system mediates pregnancy, implantation, sperm motility, sperm-oocyte interactions, the capacitation process, and embryo development [42]. The focal adhesion pathway and chemokine signaling pathway displays significant differences according to live birth outcomes of the patients. There is a gap junction-dependent interaction between GCs and oocytes [43]. The chemokine signaling pathway plays an active role in the regulation of the cumulus extracellular matrix to promote successful fertilization by stimulating integrin attachment to extracellular matrix proteins [44]. According to our results, neurotrophic factor pathways and transforming growth factorbeta (TGF-β) pathways seem to be correlated with patients’ live birth outcome. A family of neurotrophic factors is expressed in ovarian follicles as antiapoptotic factors that promote the developmental competence of oocytes [45]. The TGF-β superfamily is a diverse group of proteins with critical roles in various physiological processes, and the members of this superfamily include several subfamilies, such as the bone morphogenetic protein (BMP) subfamily and anti-Müllerian hormone (AMH; also termed as Müllerian-inhibiting substance). These are expressed in the ovary and regulate folliculogenesis [46-48]. The results of our recent study also demonstrate that there is a significant difference in the expression of BMP2 in the CCs of goodquality oocytes, and subsequently good embryos [49]. CCs associated either with developmentally incompetent or competent antral oocytes have been compared using microarrays with the whole transcriptome, and increased AMH gene expression has been shown to be correlated with oocyte competence [47]. Using gene expression profiles with microarray techniques, it is thought that CCs could have the potential to be used as a biomarker to predict oocyte quality and the pregnancy outcome of an embryo [1,50].
Although it was not as significant as in the clinical pregnancy analysis, circadian entrainment is also found to be significantly altered in the live birth analysis. Circadian entrainment ranks 18th among the most significantly altered pathways in the live birth analysis, whereas it ranks first in the clinical pregnancy analysis. This striking difference between the role of circadian entrainment in clinical pregnancy and in live birth may have resulted from the fact that different genes and interactions are significantly altered in the circadian entrainment pathway in the live birth analysis in comparison to the clinical pregnancy analysis. These altered genes and interactions can be seen in Figure 2.
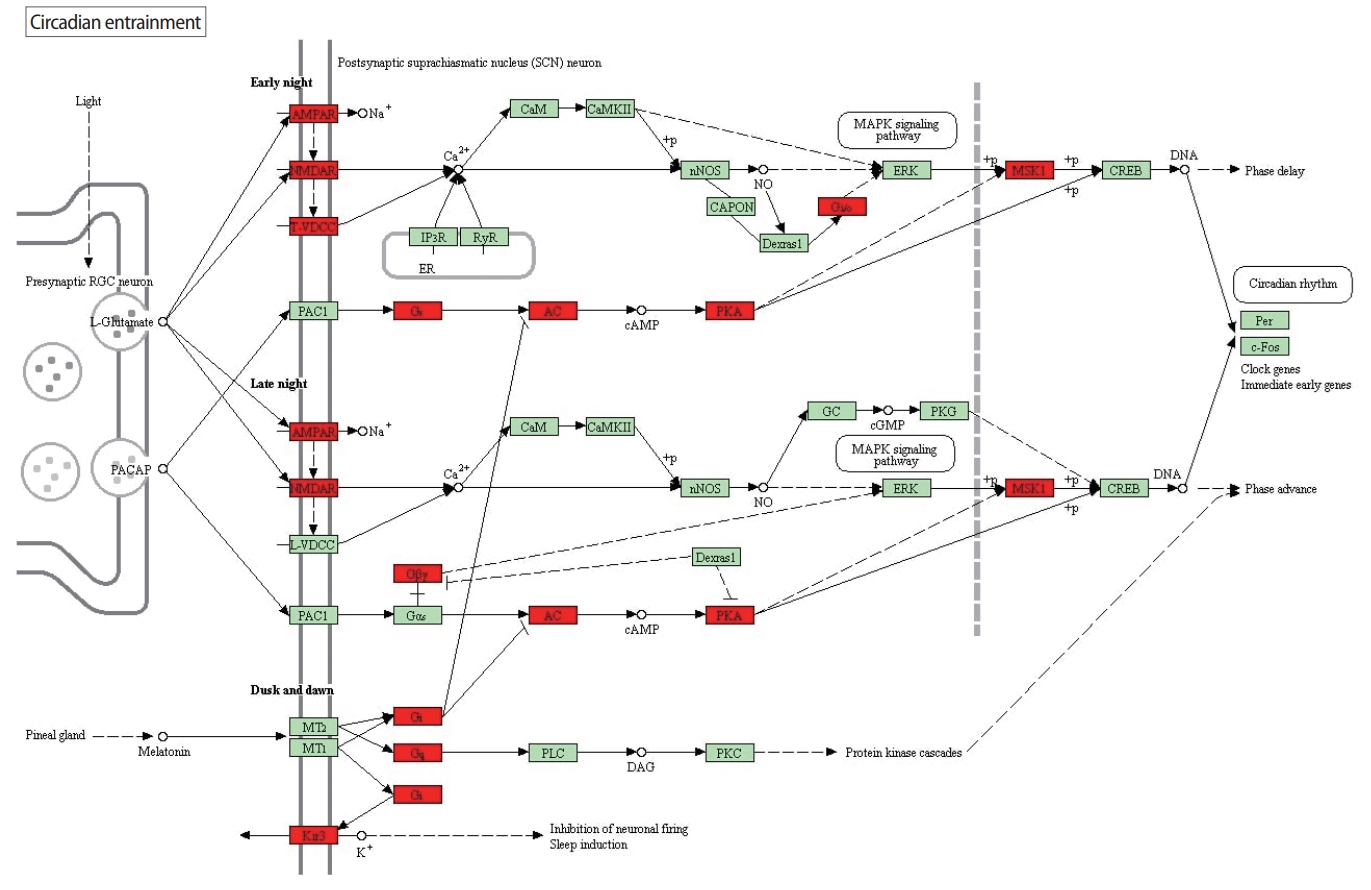
Significantly altered genes in the circadian entrainment pathway and their interactions for live birth, denoted by red pathway nodes. Data from Kanehisa Laboratories. It is permitted to be used for academic purposes. Full definitions and descriptions for the pathway nodes can be found at https://www.genome.jp/kegg-bin/show_pathway?map=hsa04713&show_description=show.
Circadian entrainment has lower p-value (6.04×10–30) than the proteoglycans in cancer pathway, which has a p-value of 1.29×10–24. This discrepancy may have occurred because 30 genes are associated with circadian entrainment pathway, whereas 17 genes are found in the proteoglycans in cancer pathway. Furthermore, the genes in the circadian entrainment pathway are generally connected to differentially expressed genes with higher significance than is the case for the genes in proteoglycans in cancer pathway. This shows that affected genes in the circadian entrainment pathway is part of many active subnetworks supporting the significance of that pathway. Overall, both pathways have relatively low cumulative average distances, supporting the fact that on average, in PPI, genes associated with significantly affected pathways are found in close proximity to the significantly altered genes found in the differential expression analysis.
In this study, significant differences are observed in the genes related to several pathways depending on the outcomes of clinical pregnancy and/or live birth. These results shed light on cellular factors that may play crucial role in clinical pregnancy and live birth. However, these results must be supported by further studies, and results may differ between patients and cycles. Nonetheless, the results of this study suggest that these pathways, especially the circadian entrainment pathway in CCs, may play essential roles in clinical pregnancy and live birth.
In conclusion, the results of this study provide new insights into human CCs and new information on the crosstalk between different signaling pathways during pregnancy. Due to their ethical advantages, current methods and those of similar noninvasive studies using CCs are of great importance. These results may further enhance the reliability of oocyte and/or embryo selection criteria and could promote the maintenance of excellent clinical outcomes in in vitro fertilization (IVF)/ICSI programs. These findings will be of value for further studies conducted to improve the success of IVF/ICSI programs.
Notes
Funding
*This work was supported by the University Scientific Research Project Fund (EgeBAP TIP-00014, 2011).
Conflict of interest
No potential conflict of interest relevant to this article was reported.
Author contributions
Conceptualization: SBD, GO. Data curation: SBD, ENTG, ET, NC. Formal analysis: HOS, US. Funding acquisition: SBD, GO. Methodology: SBD, OY. Project administration: SBD, GO, OY. Visualization: SBD, HOS. Writing – original draft: SBD, US, HOS. Writing - review & editing: SBD, US, HOS.
Acknowledgements
We would like to thank Duygu Unuvar Purcu (Biologist) for technical help in some experiments.